Optimizing Multi-Modal Fixed Fleet Transit Operations
Public transportation infrastructure is an essential component in cultivating equitable communities. However, public transit agencies have historically struggled to achieve this since they are often severely stressed in terms of resources as they have to make the trade-off between concentrating service into routes that serve large numbers of people and spreading service out to ensure that people everywhere have access to at least some service. In this project we are working on improving the efficiency of the operation by designing transit operation algorithms and real-time data analysis. Our approach focuses on taking an integrated approach to system-wide optimization, (the microtransit, fixed line and paratransit) focusing on three objectives: minimizing energy per passenger per mile, minimizing total energy consumed, and maximizing the percentage of daily trips served by public transit. While it is possible to optimize these decisions separately as prior work has done, integrated optimization can lead to significantly better service (e.g., synchronizing flexible courtesy stops with microtransit dispatch for easy transfer). However, this is hard due to uncertainty of future demand, traffic conditions etc. We address these challenges using state-of-the-art artificial intelligence, machine learning, and data-driven optimization techniques. Deep reinforcement learning (DRL) and Monte-Carlo tree search form the core of our operational optimization, which is supported by data-driven optimization for offline planning and by machine learning techniques for predicting demand, maintenance requirements, and traffic conditions. Our hypothesis in the project is that the integration of data-driven methods with better operational research methods combined with a socially engaged design will lead to success.
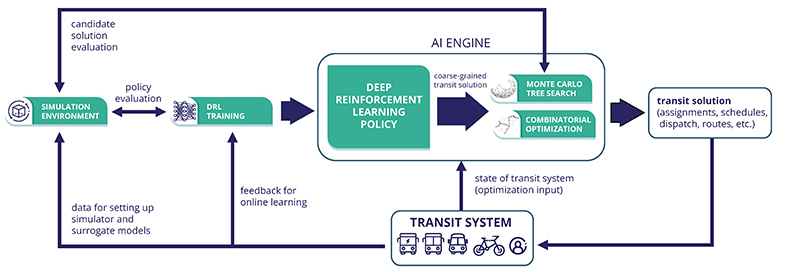
Figure 1: Integrated System Wide Optimization Approach
This research effort is part of the broader research that is being conducted in the area of smart and connected communities (SCC). As a research area, SCC is multidisciplinary and lies at the intersection of cyber-physical systems, data science, and social sciences. This research area is enabled by the rapid and transformational changes driven by innovations in smart sensors, such as cameras and air quality monitors, which are now embedded in almost every physical device and system we use, from watches and smartphones to automobiles, homes, roads, and workplaces. Coupled with emerging new modes of networking, new algorithms for data analytics, and new paradigms of distributed computing like fog computing, these sensors create an “Internet of Things” (IoT) that provide endless opportunities for innovation and improving the quality of life, such as improved transportation with reduced congestion and more efficient use of energy and water. The effect of these innovations can be seen in a number of diverse domains, such as transportation, energy, emergency response, and health care, including the transit-related efforts of our team.
Read more at the National Science Foundation page.
Website: https://smarttransit.ai/
Media Articles:
- https://news.vanderbilt.edu/2020/09/17/vanderbilt-researcher-receives-3-9-million-in-grants-to-redesign-regional-transit-system-using-artificial-intelligence-community-engagement/
- https://engineering.vanderbilt.edu/news/2018/vanderbilt-engineers-to-train-neural-networks-and-enhance-chattanooga-transit-system/
- https://news.vanderbilt.edu/2020/07/10/vanderbilt-researcher-optimizing-public-transit-with-artificial-intelligence/
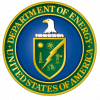
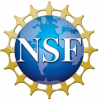