Collaborative Research: CPS: TTP Option: Medium: Coordinating Actors via Learning for Lagrangian Systems (CALLS)
This project will improve the ability to build artificial intelligence algorithms for Cyber-Physical Systems (CPS) that incorporate communications technologies by developing methods of learning from simulation environments. The specific application area is connected and automated vehicles (CAV) that drive strategically to reduce stop-and-go traffic.
This project will develop new reinforcement learning approaches for Lagrangian control that accommodate communication and networking between actuators. A motivating domain that will be an application area of the project is CAVs. A major challenge is leveraging a small number of CAVs before those technologies realize full adoption rates. Vehicle and infrastructure communication technologies can be more useful for congestion management when feeding into a group of sparse, coordinated Lagrangian control agents.
A major challenge in utilizing reinforcement learning and other advanced AI approaches at a societal scale is building a simulation that accurately captures the complexity of the system in question, and how it can be controlled.
The project includes partners from Toyota and Nissan that support testbeds enabling the research and accelerate transition of research to practice. The project also includes state and local Government stakeholders / partners which will facilitate experimentation in the real-world and demonstration of traffic congestion objectives as well as potentially emission reduction. Tools, technologies, and datasets generated in this project will be shared as active resources to support access beyond the life of the project. The project brings a focus on mentorship for undergraduate researchers, in order to broaden participation in computing.
Employing communication between vehicles can improve the efficiency of vehicle control systems to manage traffic compared to vehicles without communication. The research of this project will explore the simulation of CAVs and how we can improve their algorithms to reduce traffic congestion, with core technology developments that are applicable to homes, health, and smart and connected communities. Increasingly at the heart of CPS are artificial intelligence algorithms, which can be programmed using a simulation of how the system should operate in the real world.
The project will use data from existing traffic sensors and testbeds to drive learning and control development. A fleet of instrumented and controllable passenger vehicles will be used for data collection and actuation. Validation experiments will be conducted using these vehicles on live roadways, and the results will be validated using a camera-based testbed that collects detailed traffic data.
The project had a unique opportunity to deploy communicating vehicles in a 100-car test in November, 2022, which were able to validate and test many different modalities of communication over LTE. The ability to deploy these vehicles and examine infrastructure changes that could scale potential distributed control over existing phone networks is a capacity not found anywhere else in the world.
Our prototype system was fielded on the open-road test in November 2022, and consists of off-the-shelf technology that, when coupled with an existing phone of a vehicle operator, may cost no more than $500 to produce, and could be significantly cheaper if integrated into vehicle electronics rather than an add-on component. When compared to previous coordinating layers for inter-vehicle communication, this is potentially a 10x reduction in cost compared to those high-frequency/low-latency communication tools, while still providing a layer by which the existing communication network can be combined with local vehicle sensors.
Results from the 100-vehicle test will be published after analysis of the gathered data.
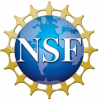